INTRODUCTION
Malaria (meaning “bad air”) is an infectious and life-threatening disease caused by a parasite (plasmodium) which is transmitted from human to human by the bite of infected female Anopheles mosquitoes, usually found in the tropical and sub-tropical regions (WHO, 2011). When the culprit mosquito sinks its sting into a person’s skin, the parasite is injected and circulated in the blood resulting in malaria fever (Eesha, 2011). The parasites multiply inside the red blood cells, which then break open within 48 to 72 hours, infecting more red blood cells. The first symptoms usually occur between 10 days to 4 weeks after infection, though they can appear as early as 8 days or as long as a year after infection. The symptoms occur in cycles of 48 to 72 hours. Malaria can also be transmitted from a mother to her unborn baby (congenitally) and by blood transfusions. In some areas of the world, mosquitoes that carry malaria have developed resistance to insecticides. In addition, the parasites have developed resistance to some antibiotics. These conditions have led to difficulty in controlling both the rate of infection and spread of this disease.
Clinical judgment for the diagnosis and management of man’s diseases is an art. It can neither be acquired from textbooks alone, nor can it be taught, but has to be developed slowly through years of observation and experience. This is because unlike other professions, which thrive on calculations based on yes/no or present/absent, very little is clearly black and white in clinical medicine. Most clinical scenarios present in shades of gray. Instead of “present or absent”, patients’ symptoms are described using terms like “never, rarely, sometimes, often, most of the times, always, etc”. Moreover, each specific symptom may also be graded as “mild, moderate or severe”. This is compounded by the fact that most symptoms are experienced and described differently by patients and many symptoms may overlap in the same patient. Each individual patient may also have a multitude of characteristics other than the disease, rendering it unique in itself. Medical problems, therefore, cannot be generalized and analyzed using imagination. An analytical program is desperately required to integrate this complex network of problems and devise individualized solutions.
MOTIVATION
According to the World malaria report 2011, malaria remains one of the world’s most deadly infectious diseases and greatest menace to modern society in terms of morbidity and mortality. There were about 216 million cases of malaria (with an uncertainty range of 149 millionto 274 million) and an estimated 655 000 deaths in 2010 (with an uncertainty range of 537 000 to 907 000). Most deaths occur among children living in Africa where a child dies every minute from malaria. Malaria is preventable and curable. Early and accurate diagnosis of malaria is essential for effective and life-saving treatment. Fuzzy logic is the nearest response to this call. It has the potential of combining human heuristics into computer-assisted decision making and provides a simple way to arrive at a definite conclusion from vague, imprecise and ambiguous medical data. Simply put, it makes the diagnosis of this deadly disease much easier, faster, and more accurate.
LITERATURE REVIEW
Today, approximately 40 percent of the world’s population- mostly those living in the world’s poorest countries – is at risk of malaria (Sola et al 2010). This makes the early diagnosis of malaria crucial as it reduces the morbidity and mortality rate of this disease. In Nigeria for instance, on the average, each Nigerian suffers at least two or more attacks every year. While millions recover, hundreds of thousands are not so lucky. This single disease accounts for about 60 percent of outpatient visits and 30 percent of hospitalizations; 25 percent of deaths in children under one year old; and 11 percent of maternal deaths —a heavy burden on Nigerian families, communities, health system, and workforce (Sola et al 2010).
Intelligent systems have become vital in the growth and survival of the healthcare sector. A good number of expert systems have been developed to manage tropical diseases. Adekoya et al (2008) developed an expert system on tropical diseases to assist paramedical staff during training and in the diagnosis of many common diseases presented at their clinics. The system was flexible, friendly, and usable by people without much background in computer operations. The study concluded that the implementation of the system reduced doctors’ workload during consultation and eased other problems associated with hospital consultations. A fuzzy expert system for the management of malaria designed by Djam et al (2011) attempted to incorporate fuzzy techniques and develop a fuzzy expert system for the management of malaria. Here, the study revealed that the use of fuzzy logic for medical diagnosis provides an efficient way to assist inexperienced physicians to arrive at the final diagnosis of malaria more quickly and efficiently. The developed system provided decision-support platform to assist malaria and Tuberculosis researchers, physicians and other health practitioners in Tuberculosis and malaria endemic regions (Djam and Kimbi, 2011). Still in the same line of thought, Olabiyisi et al (2011) designed a decision-support model for diagnosing tropical diseases using fuzzy logic. The aim of this research was to detect the disease of a patient based on the patient’s complaints and also the level of severity of the patient’s complaints. In another related work, “cognitive analysis of multiple sclerosis utilizing fuzzy cluster means” designed by (Imiavan and Obil 2012), neuro solutions and crystal reports were used for neural network analysis and graphical representation to aid in the diagnosis of multiple sclerosis, which eliminates the challenges posed by the shortage of medical experts. Several publications have successfully explained the benefits and challenges in using expert system for medical diagnosis.
TYPES OF MALARIA
According to Eesha (2011), there are four types of malaria that are known to infect man. These are
- Plasmodium Vivax (P.V.): It has the widest distribution around the globe. Approximately 60% of infections in India are caused by P.V. Although it seldom causes death or other serious problems, it can still cause major illness. Some of the common symptoms of P.v are fatigue, diarrhea, fever and chills.
- Plasmodium Ovale (P.O): This is the rarest of all the malaria types and is mostly found in Ghana, Liberia, Nigeria and the tropical West African region. Plasmodium ovale reoccurs in some cases because the parasite can rest in the liver of a patient for a few months to 4 years after getting infected by the mosquito-carrying malaria. These parasites are most likely to relapse and invade Red Blood Cells (RBCs) and make the patient sick again.
- Plasmodium malariae (P.m): This type of malaria is not as wide spread as the other types and is known to have less than 1 percent infections in the Indian subcontinent. The tropical and subtropical regions of South and Central America, South East Asia and Africa have been witnessing its effects for a long time. Although it is not lethal, it still has varied distributions and is ranked third in prevalence. It manifests itself in the form of high fever and chills.
- Plasmodium falciparum (P.f): The plasmodium parasite is recognized as the most lethal parasite that causes most infections and deaths related to malaria. This type of malaria is found in Africa, South America, and South East Asia. The individual infected by this parasite experiences fatigue, dizziness, abdominal pain, aching muscles, sore back, joint pain, vomiting, nausea, fever, headache, anaemia and some neurological symptoms as well. Since it is the severest of all the four malaria types, it becomes important that this be checked, diagnosed and treated on time. This infection also has an adverse affect on brain and the central nervous system. Many times, changes in the levels of consciousness, paralysis and convulsions can also occur.
MALARIA SYMPTOMS
In this work the following malaria symptoms were considered;
- Fever,
- Headache,
- Nausea,
- Vomiting,
- Enlarge liver,
- Joint pain,
- Dizziness,
- Abdominal pain
- Jaundice
- Anemia
- Body weakness
FUZZY LOGIC
Fuzzy logic is the science of reasoning, thinking and inference that recognizes and uses the real world phenomenon that everything is a matter of degree (Beth et al, 2002), (Parvinder et al, 2008)). In the simplest terms, Fuzzy Logic (FL) is a branch of machine intelligence that helps computers paint pictures of uncertain world (Imianvan and Obi, 2012). It presents an inference morphology that enables appropriate human reasoning capabilities to be applied to knowledge-based systems. The theory of fuzzy logic encompasses a mathematical strength to capture the uncertainties associated with human cognitive processes. Fuzzy logic is a methodology that captures and uses the concept of fuzziness in a computationally effective manner (Lofti,1994). This concept was developed over 36 years ago when Lofti Zadeh, originally an engineer and systems scientist, expressed the concern that as the complexity of a system increased, the information afforded by traditional mathematical models rapidly declined. He felt this was due to the way in which the variables in the system were represented and manipulated in a binary manner: variables (for instance, drug response) would be defined in discrete terms (that is, poor or good). Using a fuzzy approach, the transition between terms can be gradual, and the binary, all or none, options become the extreme ends of a continuum. The fuzzy view of the world was put into operation for computational purposes through the use of fuzzy sets. Fuzzy sets Variables, variable terms, and definitions can be thought of in terms of sets and set theory (Oduguwa et al 2007). In traditional set theory, using a binary view, something either belongs to a set or does not, depending on whether it fits the definition for that set. In other words, it has a degree of membership (μ) to the set either equal to one (μ=1) or equal to zero (μ=0). In fuzzy set theory, something can partially belong to a set. A value for a variable might partially belong to a set and have a degree of membership anywhere between zero and one (i.e. 0 ≤μ≤1), and thus it can partially belong to several sets with the total membership adding to one (Box 1).
Although often represented as trapezoidal, fuzzy sets can have different shapes including triangular, sigmoidal, bell shaped or irregular (Beth et al. 2002). Recently, the concept of Type II fuzzy sets has been introduced, in which the borders of the fuzzy sets are represented as upper and lower boundaries rather than as one line, in order to better express fuzziness (Ademola, 2007), (Mehdi et al, 2010). It is fuzzy sets, their definitions and relationships that form the basis of fuzzy system modeling.
There are two main characteristics of fuzzy systems that give them better performance for specific applications: 1)Fuzzy systems are suitable for uncertain or approximate reasoning, especially for the system with a mathematical model that is difficult to derive. 2)Fuzzy logic allows decision making with estimated values under incomplete or uncertain information (Cengiz et al, 2006), (Shirazi et al, 2005).
In particular, Fuzzy Logic allows us to use linguistic variable to model dynamic systems. These variables take fuzzy values that are characterized by a label (a sentence generated from the syntax) and a meaning (a membership function determined by a local semantic procedure). Fuzzy Logic is one of the branches of fuzzy set theory. Other branches of fuzzy set theory are fuzzy arithmetic, fuzzy mathematical programming, fuzzy topology, fuzzy graph theory and fuzzy data analysis.
FUZZY SET
A fuzzy set is any set that allows its members to have different grades of membership function in the interval [0, 1]. Fuzzy set can be applied in genealogical research where an individual is searching a vital record. The fuzzy membership function µA(x) indicates the degree of belonging to some element x of the universal set X. fuzzy set maps each element of x to a membership grade between 0 and 1 in various shapes such as triangular, trapezoidal, sigmoid and Gaussian.
In this work, the triangular membership function is used which is calculated as follows
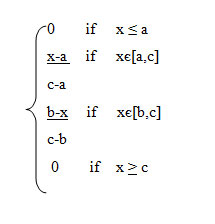
EXPERT SYSTEM
An expert system is a computer system that emulates the decision-making ability of a human expert. Expert systems are designed to solve complex problems by reasoning about knowledge like an expert, and not by following the procedure of a developer like in conventional system. To run an expert system, the engine reasons about the knowledge base like human. Figure 1.0 shows the architecture of Fuzzy expert system for Malaria diagnosis.
ALGORITHM OF THE FUZZY EXPERT SYSTEM FOR MALARIA DIAGNOSIS
- Step Input signs and symptoms of patient complaint into the system. Where m = number of signs and symptoms.
- Step Search the knowledge-base for the disease, which has the signs and Symptoms identified.
- Step Get the weighing factors (wf) (the associated degree of intensity) wf = 1, 2, 3, 4 Where 1 = low, 2 = average, 3 = high, 4 = Very high.
- Step Apply fuzzy rules.
- Step Map fuzzy inputs into their respective weighing factors to determine their degree of membership.
- Step Determine the rule base evaluating (non-minimum values).
- Step Determine the firing (conclusion) strength of the rules R.
- Step Calculate the degree of truth R, of each rules by evaluating the nonzero Minimum value.
- Step Compute the intensity of the disease.
- Step Output fuzzy diagnosis.
KNOWLEDGE BASE
Knowledge is a key factor in the performance of intelligent systems. Knowledge base is a special kind of database for knowledge management which provides a means for information to be collected, organized, shared, searched and utilized. In another term, the knowledge base acts as a repository for information in the expert system. The knowledge base of the fuzzy expert system for the diagnosis of malaria is composed of structured information. The structured knowledge is concerned with facts, rules and events of tropical disease and fuzzy rules which will be used to determine the rate of the disease.
FUZZIFICATION
This is the process of transforming crisp input into linguistic variables using the membership function in the fuzzy knowledge base. There are three types of fuzzifiers: the trapezoidal fuzzifier, triangular fuzzifer, and Gaussian fuzzifier. This work concentrates on using triangular fuzzifer for changing of scalar value to fuzzy set which is in the range of 0 and 1. In another term fuzzification canbeviewed as the operation of transforming a crisp set to a fuzzy set, or a fuzzy set to a fuzzier set.” The crisp input (that is, the measured value) is translated into linguistic variable
To obtain the degree of symptom, we use the formula:
Xi/ Xn ———————— eq (1)
Xi = number of the linguistic variable
Xn = the total number of linguistic variables
This formula is used to prepare the triangular fuzzy table. For instance if a patient complains of high headache, this is gotten as 3/4 =0.75
LINGUISTIC VARIABLES
By linguistic variables we mean variables whose values are words or sentence in a natural or artificial language. The linguistic variables used in this work are, Low, Average, High and Very High. By using these linguistic variables, fuzzy IF THEN RULES which are the main output of the fuzzy system would be set up and generally presented in the form of:
IF x is a THEN y is b. Table 1.0shows ranges of Fuzzy value
In the development of fuzzy expert systems, we construct the ranges of fuzzy value as seen in table 1.0, then we construct the fuzzy rules structure for the diagnosis of malaria as shown in Table 2.0
An interpretation of the above rules 001 to 020 are as follows
001: IF anemia = average and nausea = high and dizziness = high and Fever= very high jaundice = high and body weakness = high and enlarge liver = high and headache = high and vomiting = high and joint pain = high and loss of appetite = high THEN Malaria = very high
002: IF anemia = average and nausea = low and dizziness = high and fever = high and jaundice =low and body weakness = high and enlarge liver = low and headache = average and vomiting = low and joint pain = low and loss of appetite = high THEN Malaria = high
003: IF = anemia = average and nausea = low and dizziness = high and fever = average and jaundice = low and body weakness = average and enlarge liver = low and headache = low and vomiting = low and joint pain = low and loss of appetite = average THEN Malaria = Average
004: IF anemia = low and nausea = low and dizziness = high and fever = low and jaundice = low and body weakness = low and enlarge liver = low and headache = low and vomiting = low and joint pain = average and loss of appetite = low THEN Malaria = Low
005: IF anemia = average and nausea = average and dizziness = very high and fever = low and jaundice = low and body weakness = average and enlarge liver = average and headache = very high and vomiting = average and joint pain = low and loss of appetite = low THEN Malaria = very high
006: IF anemia = average and nausea = low and dizziness = average and fever =average and jaundice = low and body weakness = average and enlarge liver = low and headache = low and vomiting = average and joint pain = average and loss of appetite = high THEN malaria = average
007: IF anemia = low and nausea = average and dizziness = low and fever = low and jaundice = low and body weakness = low and enlarge liver = average and headache = low and vomiting= average and joint pain = low and loss of appetite = low THEN Malaria = low
008: IF anemia = average and nausea = average and dizziness = average and fever = average and jaundice = low and body weakness = average and enlarge liver = low and headache = average and vomiting = average and joint pain = average and loss of appetite = average THEN Malaria = Average
009: IF anemia = average and nausea = average and dizziness = average and fever = low and jaundice = low and body weakness = high and enlarge liver = low and headache = average and vomiting = low and joint pain = average and joint pain = high and loss of appetite = average THEN Malaria = High
010: IF anemia = average and nausea = average and dizziness = average and fever = average and jaundice = average and body weakness = average and enlarge liver = average and headache = average and vomiting = average and joint pain = average and loss of appetite = average THEN Malaria = Average
011: IF anemia = low and nausea = average and dizziness = low and fever = low and jaundice = low and body weakness = low and enlarge liver = average and headache = low and vomiting = average and joint pain = low and loss of appetite = average, THEN Malaria = Low
012: IF anemia = high and nausea = very high and dizziness = very high and fever = average and jaundice = high and body weakness = high and enlarge liver = high and headache = very high and vomiting = low and joint pain = average and loss of appetite = high, THEN Malaria = Very high
013: IF anemia = average and nausea = average and dizziness = very high and fever = low and jaundice = low and body weakness = average and enlarge liver = average and headache = very high and vomiting = average and joint pain = low and loss of appetite = low THEN Malaria= Very high
014: IF anemia = average and nausea = low and dizziness = high and fever =very high and jaundice = high and body weakness = very high and enlarge liver = average and headache = average and vomiting = average and joint pain = low and loss of appetite = low THEN Malaria = very high
015: IF anemia = average and nausea = low and dizziness = low and fever = low jaundice = low and body weakness = high and enlarge liver = low and headache = low and vomiting = average and joint pain = low and loss of appetite = high THEN Malaria = High
016: IF anemia = high and nausea = average and dizziness = average and fever = high and jaundice = high and body weakness = high and enlarge liver = high and headache = high and vomiting = high and joint pain = high and loss of appetite = average THEN Malaria = very high
017: IF anemia =average and nausea = average and dizziness = low and fever = low and jaundice = low and body weakness = average and enlarge liver = low and headache = average and vomiting = average and joint pain = low and loss of appetite = average THEN Malaria = Average
018: IF anemia = average and nausea = average and dizziness = low and fever = average and jaundice = average and body weakness = high and enlarge liver = high and headache = high and vomiting = high and joint pain = average and loss of appetite = average THEN Malaria = High
019: IF anemia = average and nausea = high and dizziness = high and fever = high and jaundice = high and body weakness = high and enlarge liver = high and headache = high and vomiting = high and joint pain = high and loss of appetite = high THEN Malaria = Very high
020: IF anemia = average and nausea = average and dizziness = high and fever = average and jaundice = low and body weakness = average and enlarge liver = high and headache =low and vomiting = average and joint pain = average and loss of appetite = average THEN Malaria = Average
FUZZY INFERENCE ENGINE
An inference engine is a computer program that tries to derive answers from a knowledge base. It is the “brain” that expert systems use to reason about the information in the knowledge base for the ultimate purpose of formulating new conclusions. In fuzzy inference engine, Fuzzy inputs are mapped into their respective weighting factors and their associated linguistic variables to determine their degree of membership. The aggregation operator is used to calculate the degree of fulfillment or firing strength of a rule.
For this work, the fuzzy logical AND is used to evaluate the composite firing strength of the rules. In practice, the fuzzy rules sets usually have several antecedents that are combined using fuzzy logical operators, such as AND, OR and NOT, though their definitions tend to vary: AND simply uses minimum weight of all the antecedents, while OR uses the maximum value. There is also the NOT operator that subtracts a membership function from 1 to give the “complementary” function. The degree of truth (R) of the rules are determined for each rule by evaluating the nonzero minimum values using the AND operator. The inference engine evaluates all the rules in the rules base and combines the weighted consequences of all the relevant (fired) into a single fuzzy set. The inference engine technique employed in this research is the Root Sum Square (RSS). RSS is given by the formula

The R1/2 + R2/2 + .. + Rn /2 are values of different rules which have the same conclusion in the fuzzy rule base, that is, R = value of firing rule. RSS combines the effects of all applicable rules, scales the functions at their respective magnitudes and compute the “fuzzy” centroid of the composite area.
DEFUZZIFICATION
The defuzzification is the process of converting the fuzzy output from the inference engine to a crisp value. That is, the output gotten form the inference engine in this work using root sum square is defuzzified to get the level of the illness. The input to the defuzzification process is a fuzzy set while the output of the defuzzification process is a single number (crisp output). There are six commonly used defuzzifying methods
- Centroid of area (COA)
- Bisector of area (BOA)
- Mean of maximum (MOM)
- Smallest of maximum (SOM)
- Largest of maximum (LOM)
- Fuzzy c-means (FCM)
In this work, the centroid of area also called center of area or center of gravity technique is used for defuzzification. This technique was developed by Takagi and Sugeno (1985). This is the most commonly used technique because of its high level of simplicity and accuracy. The centroid defuzzification technique can be expressed as

where x* is the defuzzified output,
µi(x)dx is the aggregated membership function and
x is the output variable i.e. center of membership function.
Research Experiment
Here, the fuzzy expert system diagnoses a patient based on the patient’s complain in order to give a first-hand preview of how the system works when implemented. The patient symptoms are graded with low, average, high and very high. If for instance, a patient with name JANE complains to the fuzzy expert doctor, as shown in Table 3.0. Table 4.0 shows the fuzzy rules for patient JANE
The above table shows the 20 rule base for patient JANE. For each of the linguistic variable the respective output membership function in the interval of [0,1] are computed from the possible rules using root sum square. The outputs from the inference engine are defuzzified using centriod of area also called center of gravity to obtain a crisp value.
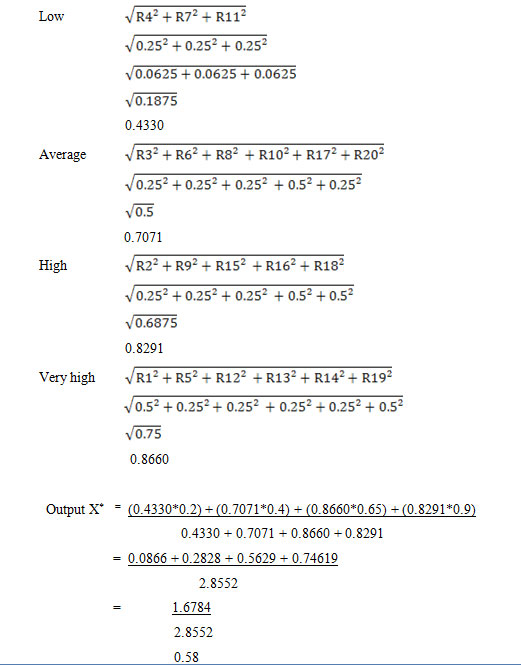
Hence the patient is having 58% malaria which is in average level using our linguistic variable
CONCLUSION
Accurate medical diagnosis is one of the major ways to sustain good health and long live. In this work, an attempt has been made to design a fuzzy expert system for the diagnosis of malaria. This is one of the best ways to deal with the vagueness and imprecision in the health care sector, and no doubt will exploit tolerance for imprecision, uncertainty and partial truth to achieve tractability, robustness, low solution cost and better report with reality in medical diagnosis of this major illness. This will in turn reduce mortality rate in cases where a limited medical doctors are available, as it provides very rapid method of diagnosis with so much accuracy and reduces the hours spent by patients in the hospital.
REFERENCES
- Ademola, O. P. (2007). Fuzzy-Wavelet Method for Time Series Analysis. PhD Thesis submitted to the department of Computing, School of Electronics and Physical Sciences, University of Surrey, Guildford, Surrey, UK
- Adekoya, A.F; Akinwale, A.T and Oke, O.E. (2008). A medical expert system for managing tropical diseases. Proceedings of the third conference on Science and National Development, COLNAS, pp 74-86
- Beth, A. S, Claudio, A. N, Burhan, I. T. (2002). Fuzzy pharmacology: theory and Applications.TRENDS in Pharmacological Sciences Vol.23 No.9 September 2002
- Cengiz Kahraman, Murat Gulbay and Ozgur kabak (2006). Application of Fuzzy sets in Industrial Engineering: A Topical Classification, StdFUZZ 201, 1 – 55. Springer-verlag Berlin Heideberg 2006.
- Eesha Duggal (2011). Types of malaria. Onlymyhealth editorial team
- Ekong, V.E., Onibere, E.A., Imianvan, A.A (2011). Fuzzy Cluster Means System for the Diagnosis of Liver Diseases. International Journal of Computer Science and Technology, IJCST Vol. 2, Issue 3, September 2011
- Djam, X. Y, Wajiga, G. M, Kimbi Y. H, and Blamah, N. V (2011). A Fuzzy Expert System for the Management of Malaria. International Journal of Pure and Applied Sciences and Technology ( 5(2) (2011), pp. 84-108
- Djam, X. Y and Kimbi Y. H (2011). A Decision Support System for Tuberculosis Diagnosis.The Pacific Journal of Science and Technology, Volume 12. Number 2. November 2011.
- Imianvan, A. A and Obi J, C (2012). Cognitive analysis of multiple sclerosis utilizing fuzzy cluster means. International Journal of Artificial Intelligence & Applications (IJAIA), Vol.3, No.1, January 2012
- Imianvan A.A. and Obi J.C.(2011). Diagnostic evaluation of hepatitis Utilizing fuzzy clustering Means. World Journal of Applied Science and Technology, Vol.3. No.1 (2011). 23-30
- Imianvan A. A.,Anosike U.F , Obi J. C.(2011). An Expert System for the Intelligent Diagnosis of Hiv Using Fuzzy Cluster Means Algorithm. Global Journal of Computer Science and Technology Volume 11 Issue 12 Version 1.0 July 2011
- Lotfi A. Zadeh (1994). Fuzzy Logic, Neural Networks, and Soft Computing. Communication of the ACM, march 1994, vol37, no 3, pp. 77-83
CrossRef
- Mehdi sotudeh C, Mohammad R, Majid M (2010). A novel soft computing approach to component fault detection and isolation of cnc x-axis drive system. Intelligent automation and soft computing, vol. 16, no. 2, pp. 177-191, 2010
- Oduguwa, V., Rajkumar. R., and Didier, F. (2007). Development of a soft computing based framework for engineering design optimisation with quantitative and qualitative search spaces. Applied Soft Computing, Vol 7(1), Jan. 2007. Pp.166 – 188
CrossRef
- Olabiyisi,S. O, Omidiora, E. O, Olaniyan, M. O & Derikoma, O. (2011). A Decision Support System Model for Diagnosing Tropical Diseases Using Fuzzy Logic. Afr J. of Comp & ICT. Vol 4, No. 2. Issue 2. p1-6
- Parvinder, S. S, Porush, B, and Amanpreet, Singh, B. (2008). Software Effort Estimation Using Soft Computing Techniques. World Academy of Science, Engineering and Technology 46 2008
- Shirazi, S. K., Noroozi, S., Carse B, Vinney, J and Rabbani, M. (2005). Investigation into hybrid data mining and soft computing techniques to aid to design of composite joints. COMPOSITES 2005Convention and Trade Show American Composites Manufacturers Association September 28-30, 2005 Columbus, Ohio USA
- Sola Ogundipe and Chioma Obinna (2010). Malaria: Killer at large. Vanguard Newspaper,September 26, 2010. Special Report
- Takagi Tomohiro and Sugeno Michio (1985).Fuzzy identification of systems and its applications to modeling and control. IEEE Transactions on systems, man, and Cybernetics, Vol. SMC-15, No. 1, January/February 1985.
- WHO (2011). World Malaria report. WHO media centre

This work is licensed under a Creative Commons Attribution 4.0 International License.